New computer vision technique counts large crowds
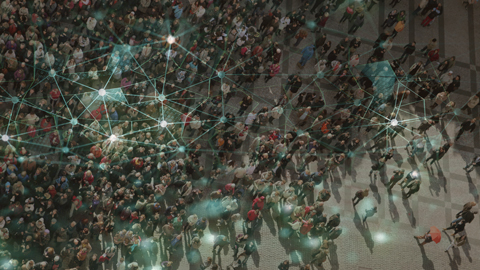
27/02/2018
Counting the number of people in large crowds in open spaces is no easy task and results can vary substantially depending on the methodologies used. Now researchers Xialei Liu and Joost Van de Weijer from the Computer Vision Centre, in a joint study with the University of Florence, have developed an algorithm which uses artificial vision techniques to estimate the number of people through images and with a 10% to 20% margin of error, the lowest ever achieved in this field. The new technique was presented at the Mobile World Congress in Barcelona last week.
This type of software is essential in the field of video security, video monitoring and behavioural analysis. Until now, the scientific problem was obvious: distortions in perspective, unequal distribution, complex lighting, variation in scales, and a long list of other elements which made the artificial vision algorithms unable to count the number of heads found in an image. The researchers from the Computer Vision Centre were able to create a stable algorithm with the use of density maps, which help to eliminate the majority of these distortions.
The technique also eliminates the greatest problem: the need to use previously processed images to train the vision algorithms. Training computers to count people in large crowds requires images previously treated by humans. The person is the one to tell the computer what each pixel contains (as when teachers show students a subject for the first time). Dr Van de Weijer and his team were able to eliminate this variable, making the process faster and less expensive. How? By showing computers to compare images.
The process is essentially very simple, but complex in practice: the computer is given an initial image, and then provided with only fragments of the same image. The computer then must learn that there are less people in the second image (fragment) than in the first (full image). This technique, after being perfected, is the learning base of the new algorithm.
Artificial vision needs an enormous amount of images in order to learn. These images are difficult to obtain, especially the ones which must be recorded and processed by humans in order to make the computers understand them. With this algorithm, Van de Weijer and his team open the door to an incredible amount of possibilities within the fields of security and surveillance, and can contribute to the open debate on the number of people at citizen mobilisations all over the world. The researchers will present this new technique at this year's prestigious Computer Vision & Pattern Recognition Conference (CVPR) taking place in June in Salt Lake City, Utah.