QuaIL, a project to power Long-Distance Communications with Quantum Physics and Machine Learning
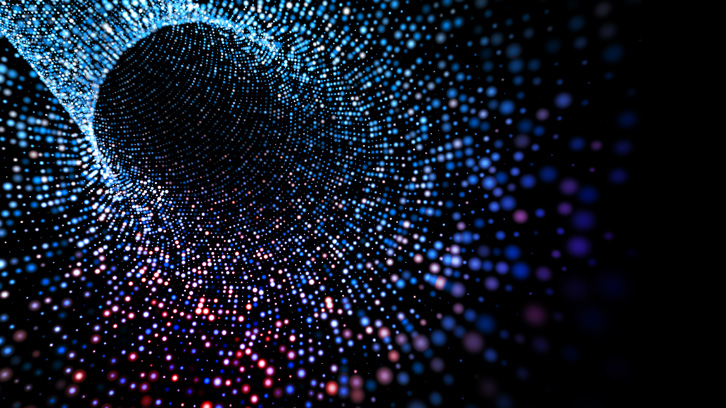
Have you ever wondered how will space-faring humans make video calls with their friends on Earth, stream their favourite TV series or communicate important messages?
Optical communications technology uses light propagating in free space and optical fiber to transmit data for telecommunications and networking. When the communication takes place over very long distances, for example between Mars and the Earth, the light signals get extremely damped and the message they transmit becomes very difficult to read. Actually, the signals are so weak that the message cannot be read without errors, even when all the technical conditions are perfectly controlled and no external sources of noise are present. This is the realm where Quantum Physics enters the game: according to Heisenberg’s Uncertainty Principle, the position and velocity of a particle cannot be measured perfectly at the same time. The same principle applies to properties of the photons, when we use them to transfer a message over very long distances.
This inherent quantum noise constitutes a new challenge for the manipulation of information that cannot be solved with classical methods. Luckily, Quantum Physics also offers us new instruments to face this kind of challenges. What is then the most efficient and reliable way to transfer huge amounts of data between Mars and the Earth? Despite many efforts of scientists in Quantum Information Theory in the past, we do not know it yet, and it might be the case that Machine Learning can help us solve the mystery.
The Marie Skłodowska Curie project QuaIL (Quantum Information Learning) will investigate the potential of Machine Learning for Quantum Communications Technologies. The research will focus on the realization of measurement devices for long-distance communications that exploit the power of quantum superposition and entanglement. Several strategies to transfer messages using photons have been developed so far, but it is still an open question how to build reliable measurement devices, or decoders, that would allow us to increase current communication rates. The project QuaIL will apply Machine Learning to create new complex decoders starting from simple quantum-optical devices that are commonly used in the lab nowadays. In doing so, the project will also investigate the connections between two promising technologies of the future, Quantum Technology and Machine Learning.
--
Matteo Rosati, the Marie Skłodowska Curie Fellow in charge of QuaIL, graduated in Theoretical Physics at Università La Sapienza, Rome, with a thesis on the modeling of Disordered and Complex Systems under the supervision of Prof. Giorgio Parisi. In 2017 he obtained a PhD in Physics from Scuola Normale Superiore, Pisa, defending a thesis titled “Decoding Protocols for Classical Communication on Quantum Channels”, under the supervision of Prof. Vittorio Giovannetti.
Since then, he has been a postdoctoral researcher at the Group of Quantum Information at UAB, working with Profs. Andreas Winter and John Calsamiglia on Quantum Resource Theories, Quantum Statistical Inference and Quantum Machine Learning.
This project has received funding from the European Union’s Horizon 2020 research and innovation programme under the Marie Sklodowska-Curie grant agreement No 845255.
Physics Department
Universitat Autònoma de Barcelona