Decision trees for data analysis on quality of life
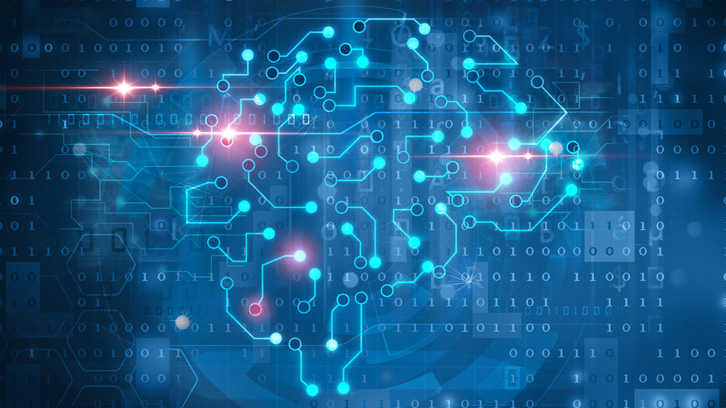
Currently, with the use of new technologies there is a great opportunity to collect data. However, the analysis of these data is difficult to do manually and this is where the knowledge discovery tools are useful. These tools allow us to obtain satisfied patterns for subsets of data, thus giving a characterization of the regularities of this subset.
One way to get these patterns is by using decision trees, which is an inductive learning method. Normally, the goal of building a decision tree is to classify new objects, but we propose to use them to analyze the data. Basically, a decision tree makes a partition of the initial data to get each subset of the partition to have only examples of one of the classes. The shape of the decision tree can give us an idea of how the database is or if we do not have examples of any of the classes.
We have used this form of analysis on two databases: one that consists of descriptions of pigs that may or may not be melanomas; and another where there are descriptions of different dimensions referring to the quality of life of people with intellectual disabilities.
At the base of melanomas we have seen that there are some parts of the domain where information is missing, since, based on the attributes that experts consider to be relevant, the tree is overfitting and very deep. In this case, then a review of how the description of the domain has been made.
In the base regarding the quality of life of people with intellectual disabilities, the situation is different. The data have been obtained from the score of surveys by social educators. This score has been discretized and, for the results obtained, it seems that the discretization intervals have not been adequate. In this case the technique has helped us to see that there is an in-depth analysis of the data and how to interpret them in order to be able to discretize them.
Thus, a decision tree of low depth and little width, means that the classes are well represented and that can be separated well because their characteristics are different. The fact that it has little depth means that with few attributes we can be able to tell which class an object belongs. On the other hand, a very deep tree means that to discriminate well between the classes many attributes are necessary, then there must be many examples to be able to make a good characterization. If the leaves of the tree have few elements (1 or 2) it means that there are very similar objects that belong to different classes. This may be due to either an error in the base (when describing any of these objects) or to the fact that with the description we have chosen for the objects of the domain we can not separate the classes correctly.
Our work has been motivated by interdisciplinary work with professionals in social education and medicine. Data analysis using artificial intelligence should always take into account that the ultimate objective is the effective improvement of people's quality of life. This fact strengthens research, where new problems, both of practical nature and theoretical nature, arise from this interaction.
Instituto de Investigación en Inteligencia Artificial (IIIA-CSIC)
Departamento de Filosofía
Universitat Autònoma de Barcelona
Eva Armengol
Instituto de Investigación en Inteligencia Artificial (IIIA-CSIC)
Àngel García-Cerdaña
Instituto de Investigación en Inteligencia Artificial (IIIA-CSIC)
Universitat Pompeu Fabra
References
Armengol E., García-Cerdaña À., Dellunde P. (2017) Experiences Using Decision Trees for Knowledge Discovery. In: Torra V., Dahlbom A., Narukawa Y. (eds) Fuzzy Sets, Rough Sets, Multisets and Clustering. Studies in Computational Intelligence, vol 671. Springer, Cham. https://doi.org/10.1007/978-3-319-47557-8_11