Automatic detection of road lines to aid drivers
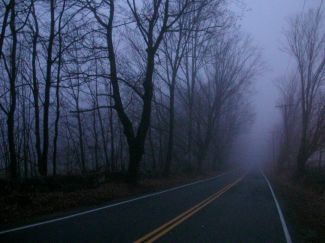
The use of cameras to detect road lines could be an affordable solution to the prevention of driving risks caused by overtaking or changing lanes. This paper proposes a system working in real-time which has given very good results. The system has been designed to identify lines in poor visibility conditions, such as during night driving or when other vehicles hinder visibility.
A present challenge of the automotive industry is to develop low cost advanced driver assistance systems (ADAS) able to increase traffic safety and driving comfort. Since vision is the most used human sense for driving, some ADAS features rely on visual sensors. Specifically, lane departure warning and lateral control can be addressed by detecting the lane markings on the road by means of a forward-facing camera and computer vision techniques. In this work we focus on this problem, which is one of the first addressed in the field of ADAS. It is a difficult and not yet completely solved problem due to shadows, large contrast variations, vehicles occluding the marks, wearied markings, vehicle ego-motion etc.
The main contributions of this work are three. The first one is to employ a different low-level image feature, namely, ridgeness, to obtain a more reliable lane marking points detection under poor contrast conditions. Aside from this practical consideration, conceptually, a ridge describes better than an edge what a lane line is: the medial axis of a thick, brighter elongated structure.
Secondly, we have adapted RANSAC, a generic robust estimation method, to fit a parametric model to the candidate lane marking points, using as input data both ridgeness and ridge orientation. Our model consists in a pair of hyperbolas sharing a common horizontal asymptote, which are constrained to be parallel on the road plane. We claim that a better suited feature (ridges) combined with a robust fitting method contribute to improve lane lines detection reliability. We have intentionally avoided any kind of result post processing, tracking or lane line prediction. Our aim has been to build a 'baseline' system to which later we can add filtering and data fusion to improve its performance.
Thirdly, we quantitatively assess the method with regard to four geometrically meaningful quantities derived from the segmented lane markings: vehicle yaw angle and lateral offset, lane curvature and width. This is possible on synthetic sequences, for which we know exactly the value for these parameters since they are provided as input to a simulator which generates the sequences. Qualitative (visual) evaluation is also performed on a number of real sequences exhibiting challenging lighting and occlusion conditions (videos).
References
"Robust lane markings detection and road geometry computation". A. Lopez, J. Serrat, C. Cañero, F. Lumbreras, T. Graf. International Journal of Automotive Technology, Vol. 11, No. 3, 2010.